MarTech Landscape: What is machine learning and why should marketers care?
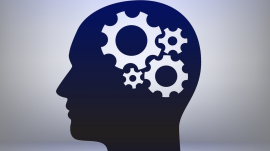

Way back in the last century, one of the most common put-downs of computers was the accusation that they only did what they were programmed to do.
Not any more.
These days, it is increasingly common for marketing and many other kinds of systems to employ some variety of “machine learning,” which moves away from the days when programmers dictated computers’ every move.
In this article, part of our MarTech Landscape Series, we look at this increasingly popular form of computing intelligence.
What is machine learning?
“Historically,” Bluecore CEO and co-founder Fayez Mohamood pointed out, “people wrote programs that were rule-based.” His company is an email and marketing personalization platform.
Now, he noted, “machine learning uses algorithms that figure out patterns in data.”
As our own Danny Sullivan reported following a Google presentation on the subject, the centerpiece of a machine learning system is the pattern-matching model that has been initially set up, against which incoming data is matched. When the match is off, the factors shaping the model are adjusted until it accurately recognizes the patterns it should. After this training period is over, the system is ready to work out in the wild.
In other words, Telerik Senior Product Manager Ivailo Ivanov told me, machine learning platforms produce insights and, directly opposing the previously mentioned 20th Century put-down, recognize patterns they were not explicitly programmed to do. Usually, these patterns or insights are delivered as recommendations to achieve certain stated goals or answer specific questions.
How is it used?
In his company’s Sitefinity content management system, for instance, machine learning might be used for something like finding “ways to get more requests for product demos” through a web site, he said. That goal is chosen from the system’s menu of possible goals.
The platform will analyze users that have requested demos, creating a model of what appears to work, and then make a number of recommendations, like suggesting blog posts about products to stimulate requests for product demonstrations. The results of that approach can then be fed back into the system, which would tune the model toward what works.
As an example goal for Bluecore’s machine learning-equipped email platform, Mohamood suggested the need to determine the number of emails you can send to specific types of customers, without having them unsubscribe. Recently, Boomtrain integrated its machine learning-based email and push notification personalization with Oracle’s Responsys marketing platform.
In fact, machine learning is becoming a common capability in so many marketing and ad tools that, in the not-too-distant future, it may be an assumed aspect of most products, like their location in the cloud is today.
Persado is using it to generate text for emails, web pages, and other marketing, and Sift Science employs machine learning to detect online fraud.
MentAd directs advertising at new prospects that best resemble your most responsive customers. And Altocloud determines if the next most effective move to get a lead or close a sale with a specific, prospective customer would be a phone call, a discount offer, or live chat.
These are among the many indicators that machine learning and more sophisticated forms of computer intelligence are dramatically changing the field, where many of the decisions that marketers used to make are increasingly being made by the platform.
Of course, the machine learning-ization of computer systems extends far beyond marketing and advertising. It includes the recent successful Google’s AlphaGo contests, the most effective timing of traffic lights, Microsoft’s touchless controller Kinect, and an improved understanding of cancer.
Image archive Shutterstock, in another example, recently announced Reverse Image Search, where users upload an image or choose one from Shutterstock’s collection, and the system employs computer vision and machine learning to find images with a similar look and feel. And, of course, there’s the “more like this” functions that show you similar Netflix movies or Amazon books.
Where is it heading?
But, while the forward arrow of machine learning is clear, the line separating it from more sophisticated arenas of artificial intelligence is continually changing.
Some people describe machine learning, and its more sophisticated cousin of “deep learning,” as forms of artificial intelligence. Others consider machine learning to be “simple” pattern matching, while AI is more involved in cognitive-like processes.
Teleriks’ Ivanov sees one difference being that machine learning generally makes recommendations, as Sitefinity does, while AI can make decisions and adaptively take action.
And Bluecore’s Mohamood describes machine learning as based on pattern-matching to achieve a goal, after training by examples, while AI is about concepts. Predictive analytics, often accompanying mentions of machine learning, models future outcomes by utilizing machine learning and other processes, including predictive modeling.
But, for marketers, the distinctions are less important than the trend.
Ivanov said that, within two to five years, he expects most platforms will not only be able to find patterns and make recommendations, but will commonly be able to adapt to new situations and challenges. In fact, IDC Research Manager Gerry Murray recently told me that, within a year, there will an explosion in the number of marketing applications that, in some fashion, “think.”
But Mohamood, who sees marketing rapidly evolving toward self-managing platforms where marketers act as goal-makers and supervisors, points to one possible limitation for computerized marketing intelligence.
If a company like fashion brand Ralph Lauren listened only to machines, he pointed out, they would only sell their popular polo shirts.
But a brand like that, he said, is more than “just about selling polos.”
From our sponsors: MarTech Landscape: What is machine learning and why should marketers care?