A case study for delivering performance in a mature Google Ads account
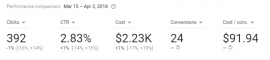

Running a Google Ads campaign can be a case of you’re damned if you do and your damned if you don’t. If the campaign turns out to be a flop your obviously in trouble, but if it turns out successful, you can run into other challenges. Campaigns that are successful over a long period can present challenges in continuing to deliver performance and add value.
In my experience, new Google Ads accounts have quick wins and low hanging fruit. Over time, these become less apparent and there is more need to innovate. One of the ways that we’ve been able to deliver performance over time on already successful accounts is through Campaign Drafts and Experiments.
In this case study, we were running a campaign for a large legal client over five years. Results were phenomenal over the period and we’d seen extraordinary growth. The Google Ads campaign was in a mature state where we were happy with performance and CPA levels, but we were challenged to continue to deliver lead growth. In this competitive industry, it was important to constantly test features and push new boundaries. For a year we ran 80 experiments to test a wide array of features. We’ll take you through some of these tests, the results we received and what we learned.
Campaign drafts and experiments
Before we do, a quick summary of campaign drafts and experiments is in order. We’ll call these “experiments” for short. This feature in Google Ads has helped us crack the issue and continue to deliver performance in a mature account. The basic process for using the tool is:
- Clone an existing campaign as a new draft
- Make desired changes within that draft to test some hypotheses
- Run this draft alongside the original campaign for some time
- Split the traffic between draft and campaign (usually 50/50) as an A/B test
- Report on results in real time throughout the test and provide updates when results are statistically significant
- Apply draft results to original campaign or reject draft campaign with a click of a button
Google has a detailed guide for setting this up which is the best resource to use as a guide
The tool has given us the freedom to rethink how we run an account and involve our clients. We can now sit down with a client and come up with a set of hypotheses that we want to test. These hypotheses are designed to align with upcoming client goals and also push performance limits. Clients are involved in a decision-making process, which is completely transparent. They were able to see the process from the formulation of the question/hypotheses through to results.
Experiments also provide a safe environment for implementing and testing new features. When account performance has been strong, we are often hesitant to rock the boat. But we still need to try out new features. Take for example the recent introduction of machine learning features and tools in Google Ads such as automated bidding strategies and responsive ads. Handing over the keys to ML algorithms can be daunting. While ML might provide incremental performance improvements, there is risk these algorithms may not perform, and then account performance will suffer. Experiments allow you to minimize these risks through testing.
What we tested
In consultation with our client, we ran an array of experiments. These were tested on a rolling basis throughout the year. As a sample, some of the key hypotheses we tested were:
- Automated bidding (maximize conversions) will provide more conversions than manual bidding.
- Automated bidding (Target CPA) will provide a better conversion volume performance than we currently achieve with manual bidding.
- A more granular campaign structure based on SKAG’s will increase the quality score for the campaign
- Responsive display ads will provide better CTR then static banners
- Responsive search ads will provide better CTR then expanded text ads
- A new landing page with less clutter will prove better conversion rates
- A new landing page with a different hero image will provide better conversion rates
- Ad copy with a question rather than statement in the first heading will provide better CTR
- Running ads at a lower position will provide a better conversion rate
- Bidding 20% higher on desktop devices only will improve conversion rate
Note that the hypotheses are specific. We are testing for only one outcome and using a specific metric to evaluate.
Aside from campaign experiments, we also ran several “ad variation experiments.” These are slightly different from campaign experiments, as they can be cross-campaign. This goes beyond the scope of this article, but we strongly recommend running these as well.
Below are the results of four experiments we ran:
1. Ad copy change example
Hypotheses: Ad copy with a question rather than statement in first heading will provide better CTR.
What we changed: Adjusted ad copy for heading one for all ads in the draft to be question-based.
Results:
Decision: This experiment ran for 18 days. The CTR increased by 1%. Results were not significant so we decided not to apply.
What we learned: There was no performance increase in having question-related ads rather than statement ads in a general sense. These needed to be adjusted on a case by case basis, based on the search query and ad.
2. Responsive search ads
Hypotheses: Responsive search ads will provide a better CTR than static search ads.
What we changed: Introduced responsive search ads into the campaign draft.
Results:
Decision: This experiment ran for 47 days. The CTR increased by 1%. Results were not significant. We still decided to apply the results, since the responsive ads did not harm performance and they were a new feature allowing us to rotate more ad copy.
What we learned: Despite not having a performance increase, we see that searchers engage well with this new ad type. We were able to minimize risk through the experiment. We continued to monitor these ad types after implementation and performance have been strong.
3. Landing page changes
Hypotheses: Adjusting the hero image on the landing page from male to female will increase conversion rates.
What we changed: Adjusted the hero image.
Results:
Decision: This experiment ran for 30 days. The conversion rate increased from 7% to 14.88%. We applied the experiment and only ran the new landing page moving forward.
What we learned: The increase in CVR was dramatic and showed that a small change, like changing the gender of the image can have a dramatic effect. We also learned that it’s likely that users respond better to female imagery in general.
4. Target CPA bidding
Hypotheses: Automated bidding (Target CPA) will provide a better conversion volume performance than we currently achieve with manual bidding.
What we changed: We set a target CPA in the draft, at the same CPA we were already achieving in the campaign with manual bidding. The hypotheses would test whether we can achieve more conversions with target CPA bidding.
Results:
Decision: This experiment ran for 34 days. The experiment achieved 53 conversions, the original campaign achieved 70 conversions and had a lower CPA as well. Therefore we decided not to implement target CPA bidding for this campaign
What we learned: Automated bidding strategies are not ideal just yet. We should add as well that the target CPA has worked better in other campaign tests we ran. We’ve spoken to Google and their recommendation was to run the target CPA draft for longer. We agree, but this is not always practical for clients with limited budgets to burn through.
Experiment considerations
As a final note, there are two key issues that are not widely discussed or reviewed in Google help articles. They are very important to consider when setting up experiments.
Having a goal in mind when generating this hypothesis is critical. You should write down in a notebook what the goal is and what metric you want to test. Defining the metric is also critical at the outset since it easy to stray from it. For example, If your testing a new ad type, then your hypothesis should probably be written in terms of CTR and not CPA. Your results might show a better CPA, but this should not sway your decision since your hypothesis is framed in terms of CTR and you should not apply the experiment!
Another way you can get a false positive result is due to time design issue. This occurs when the experimenter increases or decreases the run time of the experiment to achieve a significant or desired result. This occurs unknowingly, the experimenter doesn’t realize that they are creating a false positive. Think about it like this: if we increase the run time for another week we might get a significant result, if we increase another week further we may get a non-significant result, so altering the period to suit our needs is not a fair test. Even in well-designed university experiments, this bias occurs.
It is important to set a time frame before the experiment starts running and stick to this. To counter this, I include the end dates within the experiment title so I know when it has to end. As a rule of thumb experiments should run for at least one month. You can also try using an ab test sample size calculator If your testing for changes in conversion rate.
The post A case study for delivering performance in a mature Google Ads account appeared first on Marketing Land.
From our sponsors: A case study for delivering performance in a mature Google Ads account